We are excited to announce our feature in the most recent edition of Nature Portfolio‘s Biopharma Dealmakers discussing the advances in AI-driven drug development.
Leveraging deep omics data to tackle a global health challenge with precision
Precision medicine is widely recognized as having revolutionized cancer treatment by enabling patients to receive the therapies best suited to the specific molecular drivers of their disease—an approach that not only delivers benefits to patients in terms of improved outcomes, but also avoids wasting healthcare resources by treating non-responder patients with ‘one size fits all’ medicines.
The transformative potential of precision medicine has not, however, made such inroads in more complex, multifactorial diseases, largely because there are very few simple genetic or protein markers that define patient subgroups who respond better or worse to given treatment regimens in these complex conditions.
Instead, they require the integration of many types of omics data, from genomics and proteomics to metabolomics and phenomics—and the ability to make sense of the massive quantities of data these provide. Inspired by the decreasing costs of generating all kinds of omics data and advances in artificial intelligence (AI), MultiOmic was founded in 2021 to tackle this problem head on and bring precision medicine to bear on metabolic syndrome-derived conditions—the world’s most prevalent set of related diseases, with a huge global healthcare cost burden.
Metabolic syndrome refers to a cluster of risk factors—insulin resistance, hypertension, dyslipidemia and abdominal adiposity—that often lead to downstream chronic medical conditions including type 2 diabetes, atherosclerotic cardiovascular disease, non-alcoholic fatty liver and chronic kidney disease (Fig. 1). A complex interplay between genetic and environmental factors drives a pattern of disease manifestation, progression and chronicity that varies across different patients. Current treatment schemes aim to reduce disease manifestation and progression using a combination of lifestyle changes and pharmacological interventions—antidyslipidemic, antiglycemic, antihypertensive and antithrombotic drugs—that cost in excess of $110 billion in 2020. The benefit of these schemes is unevenly distributed across patients and seems to, at best, delay the onset of serious complications, suggesting that the patient population is highly heterogeneous and that these drugs do not modify the underlying pathophysiological processes.
Worldwide, one in two adults exhibit metabolic syndrome or suffer from its downstream diseases, decreasing quality of life and contributing to 45% of all deaths. And the burden does not only fall on patients but on healthcare systems around the world: in 2020, the cost of treating people for these chronic conditions was estimated to be $1.8 trillion—more than 1.5 times the cost of treating cancer. As populations age over the next two decades, the incidence of metabolic syndrome and its derived diseases is expected to triple, with the cost of treatment then predicted to exceed $5 trillion.
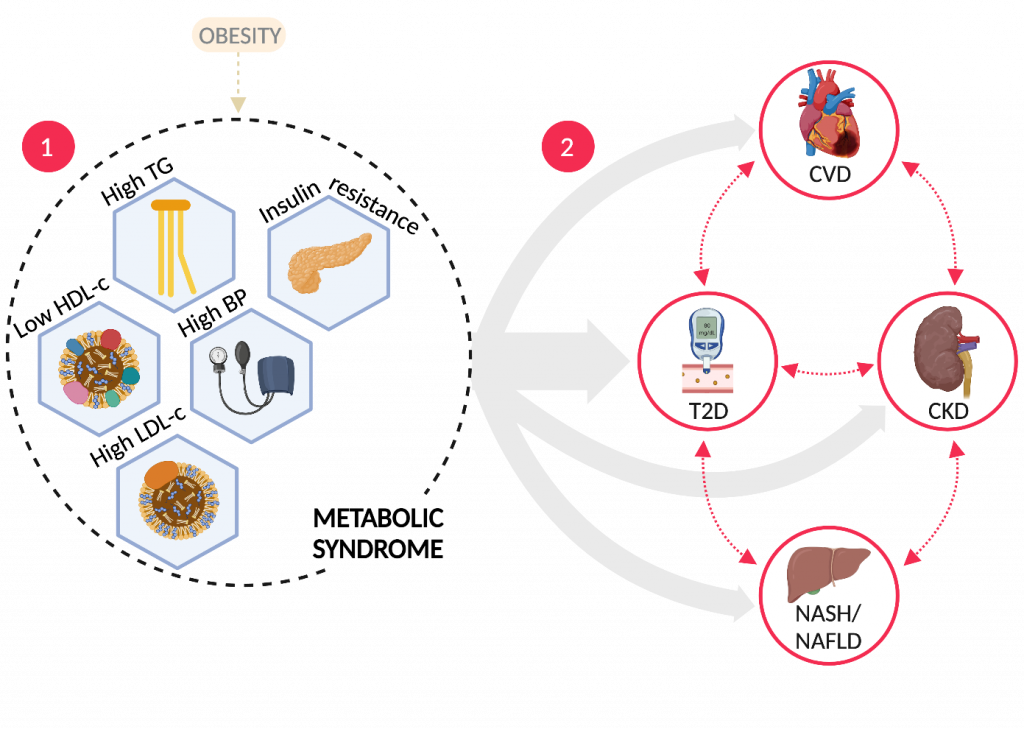
Fig. 1 | The metabolic syndrome. Metabolic syndrome is a cluster of risk factors (1) that often lead to downstream chronic medical conditions (2). Grey arrows represent different sub-populations (endotypes) with varying progression timelines and drivers. BP, blood pressure; CKD, chronic kidney disease; CVD, cardiovascular disease; HDL-c, high-density-lipoprotein cholesterol; LDL-c, low-density-lipoprotein cholesterol; NASH, non-alcoholic steatohepatitis; NAFLD, non-alcoholic fatty liver disease; T2D, type 2 diabetes; TG, triglycerides.Figure created with BioRender.com
Applying a multi-omic approach
MultiOmic aims to unravel the heterogeneity in metabolic syndrome biology using a multi-omic approach combined with deep patient phenotyping. By studying patients longitudinally along different phases of the disease continuum, MultiOmic expects to identify disease drivers in specific patient clusters, and use these disease signatures to develop precision medicine assets with the potential to transform the treatment of metabolic syndrome-related diseases, in much the same way as precision medicine gave a massive boost to oncology.
From 1990 to 2000, the cancer mortality rate declined by just 2% (having risen continuously over the previous 70 years), but from 2000 to 2019, it declined a further 15%. Since 2000, more than 80 cancer precision medicines have become available for almost 40 distinct patient sub-populations. The 5-year survival rate now exceeds 67% and an estimated 50–60% of all cancer patients can now be treated with a precision drug. Over the same 2000–2019 period, the cancer drugs market grew from $10 billion to more than $140 billion.
The initial challenge in developing precision medicines for metabolic syndrome-related conditions is identifying endotypes. An endotype is a patient sub-population with an underlying pathophysiological mechanism distinct from the wider population of patients otherwise displaying the same clinical phenotype. As MultiOmic concentrates on chronic multifactorial disorders arising from a complex interplay of inherited and environmental factors, very few endotypes in this space are likely to be defined by a single genotype. Most endotypes in this field are likely to be polyomic—identifying them will require integrating many kinds of molecular omics and phenomic data.
The MOHSAIC platform
MultiOmic is building its MOHSAIC platform (Fig. 2) to pursue this mission. The initial ‘Patient-to-Data’ component of this platform will control sample collection protocols, ingest patient samples, curate clinical information and generate huge quantities of longitudinal, proprietary multi-omic data. The Patient-to-Data journey begins with MultiOmic’s carefully selected patient cohorts from its data partners, including scientific research institutions, healthcare providers, biobanks and healthtech companies. These data partners do not directly supply molecular data; instead, they provide de-identified biological samples (such as blood, urine, or faeces) and corresponding clinical information, collected under strict protocols agreed with MultiOmic. MultiOmic is implementing a network of service providers (omics technology specialists, diagnostic laboratories, plus logistics and shipping companies) to convert these samples into the whole gamut of omics data (genomic, epigenomic, transcriptomic, proteomic, metabolomic, microbiomic) for integration with the phenomic data curated from clinical information supplied by its data partners.
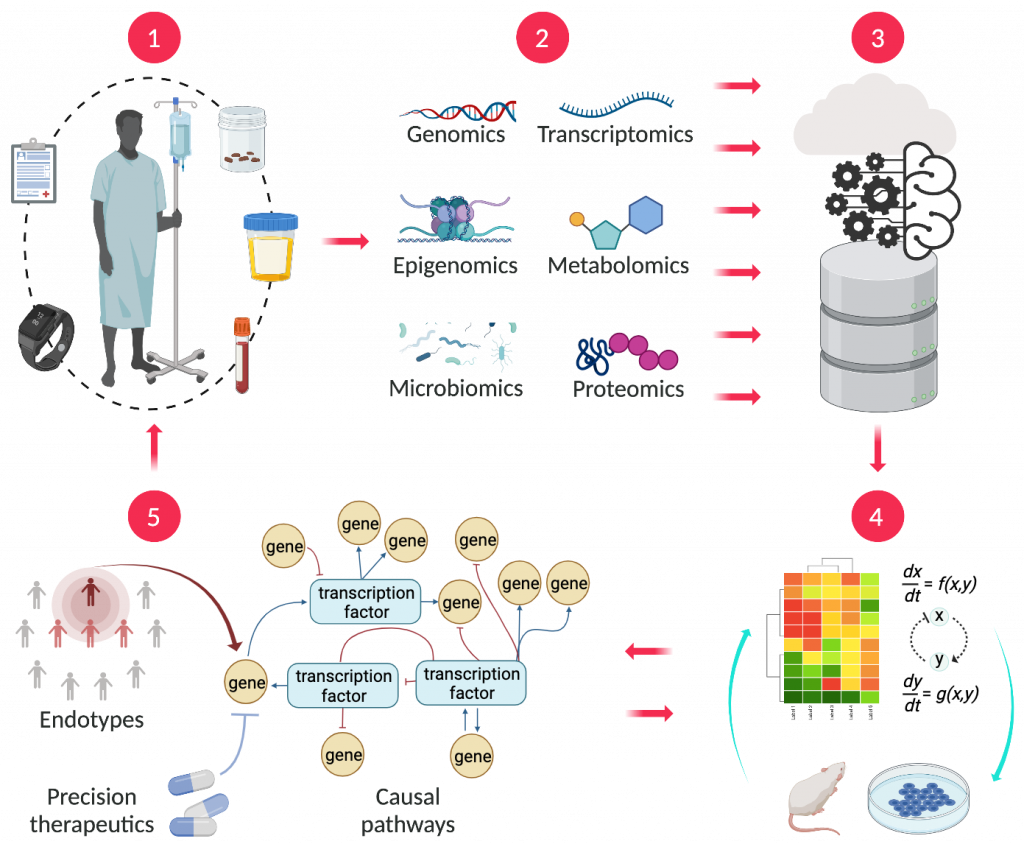
Fig. 2 | The MOHSAIC platform. MultiOmic’s process begins with the collection of biological samples along with clinical and outcome data (1). Multi-omics data derived from these samples (2) is pooled into a central data lake (3), which is used to build artificial intelligence (AI)-enabled computational system biology models that are fine-tuned with wet lab experimentation (4), to identify endotypes, causal pathways and precision therapeutics (5).Figure created with BioRender.com
Metabolic syndrome and its downstream conditions comprise a chronic patient journey that evolves over time, sometimes accelerating and sometimes slowing down in response to changes in lifestyle, medication or other factors. MultiOmic will build up a longitudinal molecular picture of the course of disease progression through biological samples taken from the same patient at different time points. By using trusted, closely monitored service providers, MultiOmic ensures the data generated from these patient samples—up to 500 Gb per patient—are consistent, standardized, and comparable. All the data will be accumulated into a single, centralized data lake that is both deep, because it combines so many types of detailed molecular omics and phenomic data, and wide, because the data derives from multiple sources accumulating to thousands of patients over multiple time points. A deep and wide disease-specific data lake is the foundation of any AI-enabled therapeutics discovery company.
The Data-to-Therapeutics component of MultiOmic’s MOHSAIC platform will then exploit this data lake, combining both data-driven and rule-based in silico modeling with bespoke ex vivo wet lab experimentation, to originate new therapeutic concepts. This process starts with data-driven modeling, using machine learning for hypothesis-free processing and exploration of the relevant portions of the data lake to identify several possible endotypes. Rule-based systems biology techniques are then deployed to build mathematical models—comprising hundreds of ordinary differential equations—of biological pathways potentially relevant to these endotypes, which are fine-tuned with data from bespoke ex vivo experimentation conducted in parallel. The resulting models are then used to prioritize one or more endotypes and elucidate the pathways underlying their pathophysiology. Simulation of pathway perturbations using the systems biology models then leads to potential therapeutic concepts for pharmacological intervention. These concepts are subsequently validated with in vitro, ex vivo and in vivo experimentation using an approach that the biopharma industry will recognize and find credible.
Potential therapeutic concepts
One example of a therapeutic concept that could emerge is a novel drug target. MultiOmic would then initiate a drug discovery program for a new molecular entity (NME) to treat certain metabolic syndrome-related indications in patients of a particular endotype, as well as a companion diagnostic development program for tools to select prospective recruits with that endotype in subsequent clinical trials. The specificity of a particular endotype enables a clinical program with smaller sample size and higher efficacy compared to traditional ‘one size fits all’ drug candidates, resulting in shorter time-to-market, lower clinical development costs and transformative patient outcomes.
Historically, clinical trials of agents for metabolic syndrome-related conditions have previously had no way of breaking out heterogeneous patient experiences into separate endotypes that respond better or worse to a given therapeutic, revealing only the average effect across all endotypes. Yet there are likely many existing drugs used for other indications—as well as drugs that have been abandoned due to lack of efficacy in metabolic syndrome-related conditions—which, although they did not perform well on average across endotypes, could show markedly better results in specific endotypes. MultiOmic aims to identify the endotypes that are most likely to respond well to these under-utilized NMEs.
Consequently, another type of therapeutic concept that MultiOmic intends to generate is repositioning an existing NME—whether already marketed, in an active clinical program or abandoned after insufficient efficacy in phase 2 or 3 clinical trials—for a specific endotype in certain indications within the spectrum of metabolic syndrome-related conditions. In a similar vein, bearing in mind the multifactorial nature of its target disease space, MultiOmic’s rule-based modeling could be used to identify combinations of existing NMEs as a third type of therapeutic concept.
Once implemented, MultiOmic will deploy its MOHSAIC platform in value-sharing partnerships with biopharma companies focused on the cardiometabolic, cardiorenal and type 2 diabetes therapeutic areas. Partnership structures envisaged include jointly funded and co-owned drug discovery programs on new targets; technology partnering/licensing arrangements to reposition the collaborator’s drug assets; and in-licensing ‘fallen angel’ NMEs for repositioning. A further commercialization route is out-licensing intellectual property to medical technology companies for developing companion diagnostic and clinical biomarkers. Finally, MultiOmic plans to collaborate with healthtech companies to develop clinical decision support systems for optimizing management of patients using existing therapeutic and intervention options.